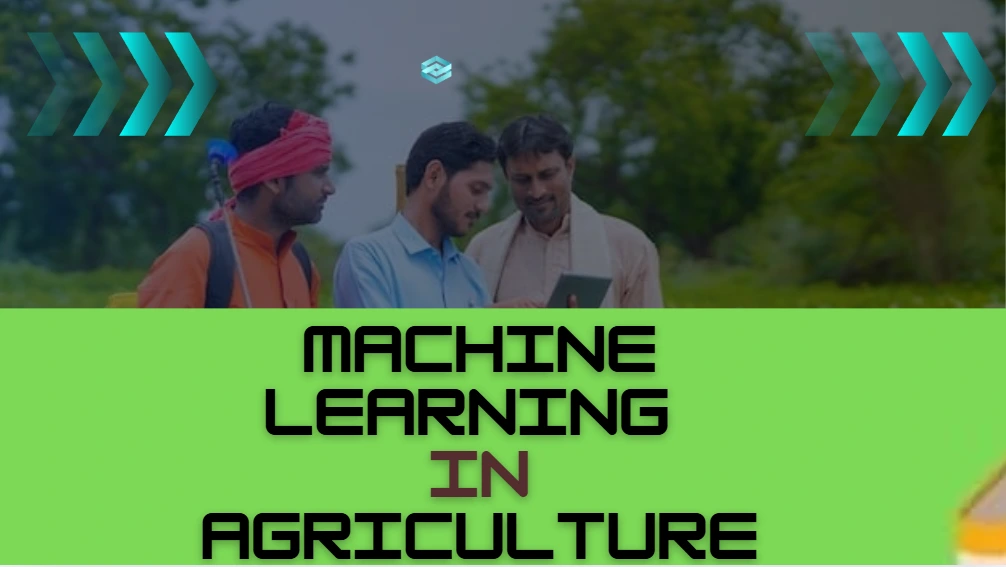
Agriculture has always been the backbone of human civilization, but with the growing global population and climate change, traditional farming methods are facing challenges. Enter Machine Learning (ML)—a branch of Artificial Intelligence (AI) that is transforming agriculture. From predicting crop yields to optimizing irrigation, machine learning is paving the way for a smarter and more sustainable future in farming. In this article, we explore the future scope of machine learning in agriculture and how it can address critical issues like food security, resource management, and environmental sustainability
What is Machine Learning in Agriculture?
Machine learning is a type of artificial intelligence. ML algorithms are trained to analyze data, identify patterns, and make predictions. In agriculture, ML provides actionable insights by collecting data from sensors, satellites, drones, and farm equipment. For example:
Crop Monitoring: Drones collect crop health data from cameras and sensors, which ML algorithms analyze to detect issues such as nutrient deficiencies or pest infestations.
Soil Analysis: ML algorithms analyze soil data to recommend the best crops and fertilizers so that crop growth is optimal.
Weather Prediction: ML models predict weather patterns and help farmers plan irrigation and harvesting, reducing the risk of crop damage due to unexpected weather changes.
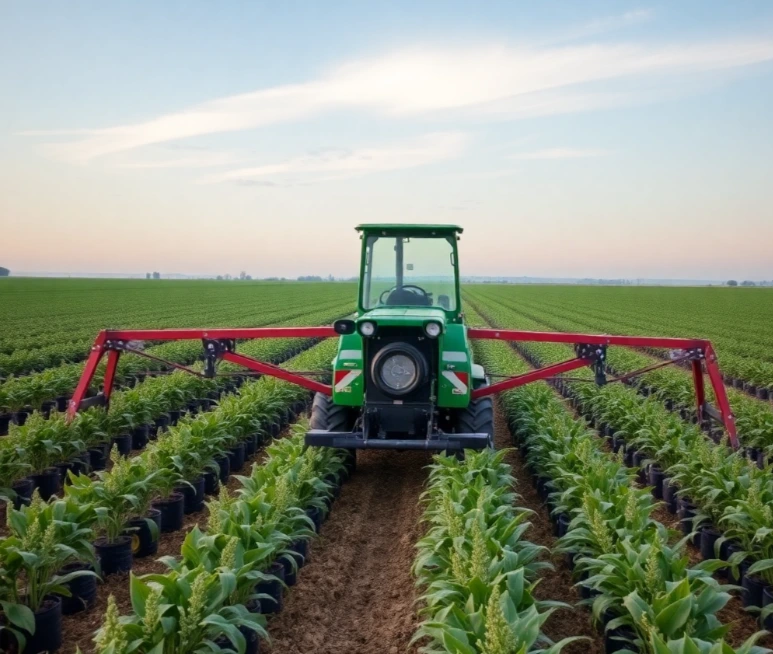
Why Machine Learning in Agriculture?
✅ Rising Demand for Smart Farming
According to a 2024 FAO report, global food demand is expected to rise by 60% by 2050, necessitating innovative solutions like ML to increase efficiency and sustainability.
✅ Government Initiatives & Support
Organizations like ICAR, NABARD, and APEDA are promoting AI and ML-based solutions in Indian agriculture through subsidies and training programs.
✅ Growth of Agri-Tech Startups
Many Indian startups, such as Fasal, CropIn, and AgNext, are using ML to enhance precision farming, pest control, and yield prediction.
Future Scope of Machine Learning in Agriculture
Top future scope of machine learning in agriculture…
Using IoT sensors and satellite imagery, machine Learning helps farmers monitor crop health, soil conditions, and weather patterns.
Example: ML algorithms analyze soil moisture, weather, and crop health data to recommend the amount of water and fertilizers needed. This not only increases crop yields but also reduces resource wastage.
Future Scope: By 2030, precision farming is expected to increase crop yields by 20% while reducing resource usage by 30%.
Revolutionizing Indian Farming: The Role of Precision Agriculture in India
Precision agriculture in india “A friend of mine, Suresh Yadav,...
Read Moreb) Crop Disease Detection
AI-based image recognition systems detect pests, diseases, and weeds, enabling early intervention and reducing pesticide use.
Example: ML models trained on images of diseased plants can identify issues like blight or rust in real-time. Farmers can then take immediate action to prevent the spread of diseases.
Future Scope: AI-powered apps will allow farmers to upload photos of crops and receive instant disease diagnoses, making it easier for even small-scale farmers to protect their crops.
c) Automated Farming Equipment
Self-driving tractors and harvesters are no longer science fiction.
Example: Companies like John Deere are using ML to develop autonomous farming machinery that can plant, water, and harvest crops with minimal human intervention.
Future Scope: By 2025, 30% of farms in developed countries are expected to use automated equipment, significantly reducing labour costs and increasing efficiency.
d) Supply Chain Optimization
Machine Learning predicts commodity prices, demand fluctuations, and logistics optimization, benefiting both farmers and agribusinesses.
Example: Predictive analytics can forecast demand for crops, helping farmers decide what to grow, how to grow, and where to grow. ML can also optimize logistics, ensuring that produce reaches markets quickly and in good condition.
Future Scope: ML will enable real-time tracking of produce from farm to table, ensuring freshness and reducing spoilage by up to 50%.
e) Livestock Monitoring & Management
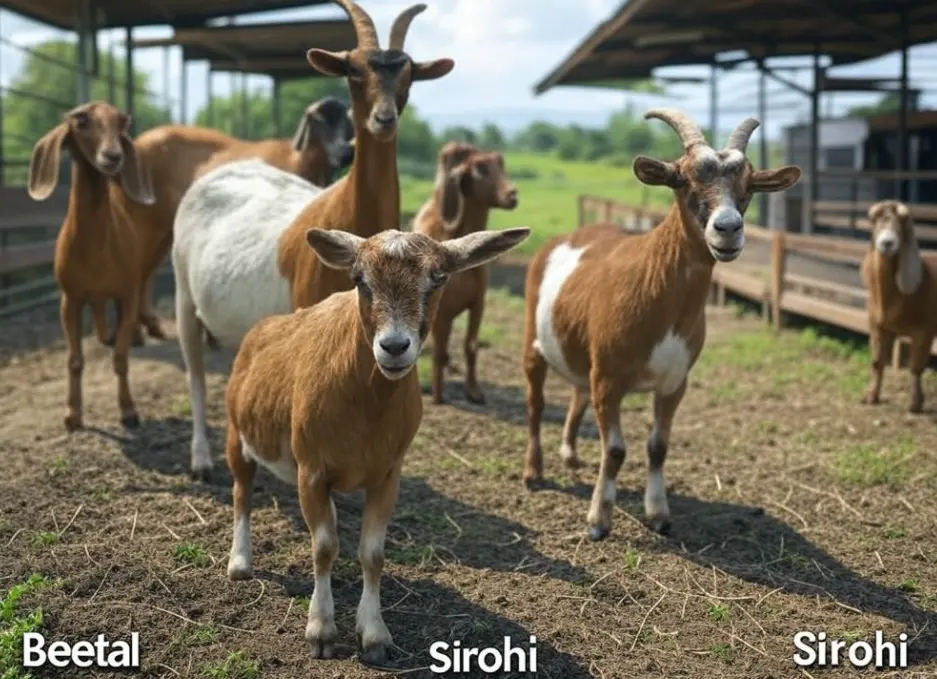
ML-powered sensors and tracking systems help farmers monitor animal health, detect diseases early, and improve livestock productivity.
Example: AI-powered cattle monitoring systems have reduced mortality rates by 30% in dairy farms.
f) Soil Health Monitoring & Fertilizer Optimization
AI-driven sensors analyze soil nutrient levels and recommend optimal fertilizer usage.
Example: AI-powered Soil Intelligence Platforms help farmers cut fertilizer costs by 20% while boosting soil fertility.
g)AI-Powered Greenhouse Automation
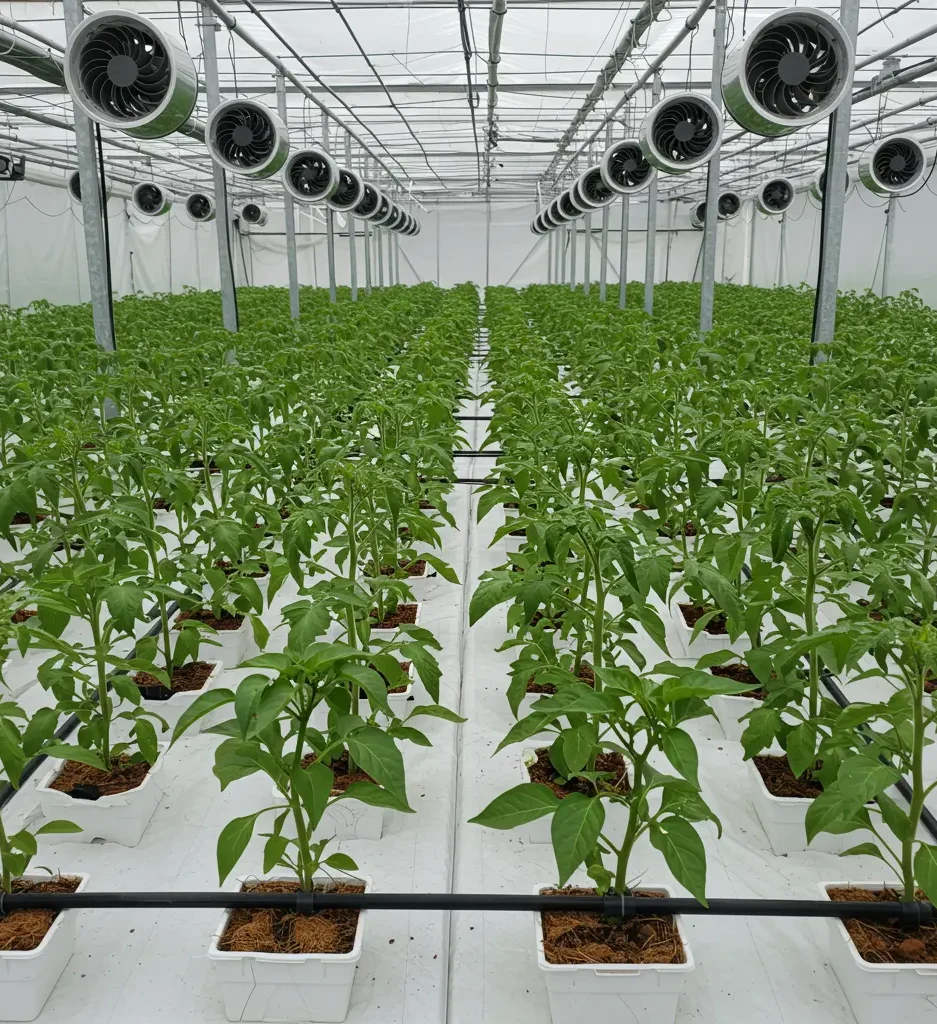
ML-based automation systems regulate temperature, humidity, and lighting to maximize greenhouse yields.
Example: Smart greenhouses using AI can increase productivity by 30%.
h) Farm Robotics & Automated Harvesting
AI-powered robots and drones are increasingly being used for seeding, weeding, and harvesting, reducing labour costs and improving efficiency.
Future Trends in ML & AI in Agriculture
a) AI-Powered Drones
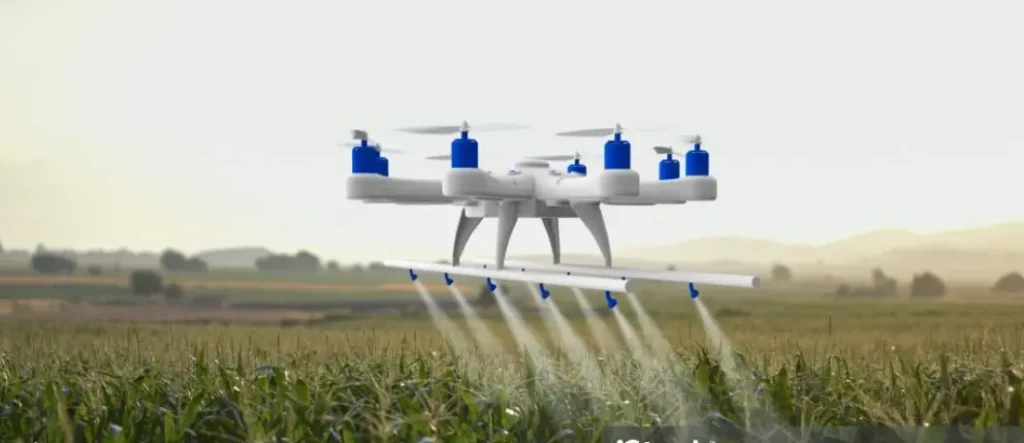
Drones equipped with AI and ML algorithms are being used to monitor crops, spray pesticides, and plant seeds.
Example: DJI’s Agras drone sprays pesticides with pinpoint accuracy, reducing chemical usage by 30%.
b) Blockchain and ML Integration
Combining blockchain with ML can ensure transparency in the agricultural supply chain.
Example: Walmart uses blockchain and ML to track the journey of produce from farm to store.
c) Vertical Farming with ML
Vertical farming, combined with ML, is revolutionizing urban agriculture.
Example: Companies like Plenty use ML to optimize lighting, temperature, and nutrient levels in vertical farms.
Benefits of Machine Learning in Agriculture
Increased Yields: ML helps farmers grow more with fewer resources, increasing crop yields by up to 20%.
Cost Reduction: Optimized resource usage reduces expenses on water, fertilizers, and pesticides.
Sustainability: ML promotes eco-friendly farming practices, reducing the environmental impact of agriculture.
Food Security: By improving crop yields and reducing waste, ML can help feed the growing global population, which is expected to reach 9.7 billion by 2050.
Challenges & Solutions in Adopting ML in Agriculture
Challenges | Solutions |
High initial investment | Govt. subsidies & startup funding |
Lack of digital literacy | Training programs for farmers |
Limited internet access in rural areas | Expansion of rural broadband infrastructure |
Data privacy concerns | Secure cloud-based ML models |
Resistance to new technology | Awareness and demonstration projects |
Real-Life Examples of ML in Agriculture
IBM Watson Decision Platform: Uses ML to provide insights on weather, soil, and crop health, helping farmers make informed decisions.
Blue River Technology: Develops ML-powered robots for weed control and crop monitoring, reducing the need for chemical herbicides.
Microsoft FarmBeats: Aims to bring AI and ML to small-scale farmers, providing them with affordable tools for precision farming.
Success Stories of ML in Agriculture
a) Story of Farmer Ramesh (Maharashtra, India)
Farmer Ramesh was worried about low crop yields due to unpredictable weather and pest attacks. After using an ML-powered farming app, he started getting real-time advice on irrigation, pest control, and fertilizer usage. His yield increased by 30% in one year, and his income doubled.
b) Precision Farming in USA
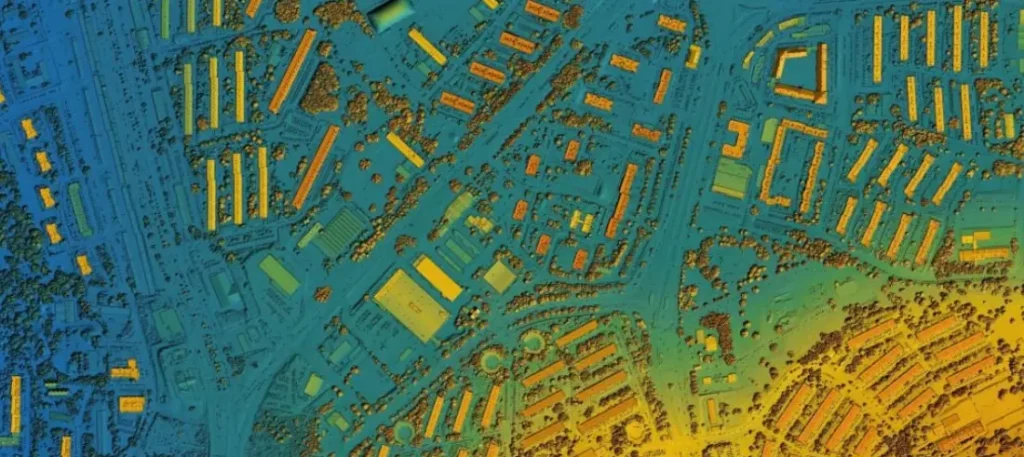
A soybean farmer in Iowa, USA optimized water and fertilizer usage using ML-based precision farming techniques. By analyzing soil and weather data, the farmer reduced water consumption by 20% and increased soybean production by 15%.
c) AI-Driven Vineyards in France
A vineyard in France used ML algorithms to monitor the health of grapes and predict the best time for harvesting. The result was a 25% improvement in grape quality and a significant increase in wine production.
How Farmers Can Adopt Machine Learning
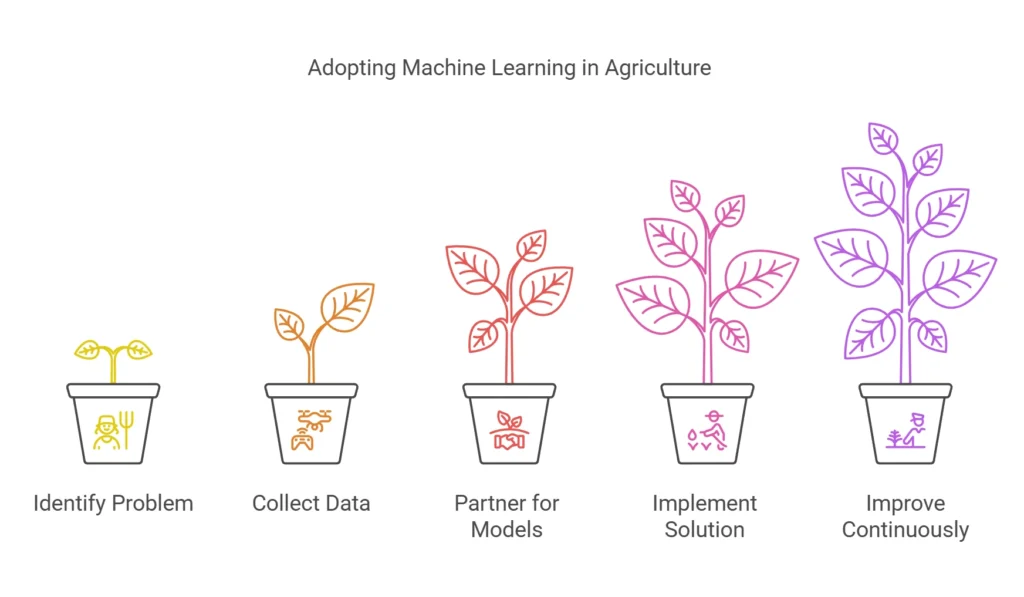
Step 1: Identify the problem (e.g., crop disease detection, irrigation optimization).
Step 2: Collect data using sensors, drones, or satellite imagery.
Step 3: Partner with tech companies or hire data scientists to develop ML models.
Step 4: Implement the solution and monitor its impact.
Step 5: Continuously improve the model based on feedback and new data.
Top 10 AI Tools for Farmers and agricultural students
Future scope of machine learning in agriculture the top 10 mobile applications, tools present in the market
IBM Watson Decision Platform
Use: Provides weather forecasts, soil analysis, and crop health monitoring.
Benefit: Helps farmers make data-driven decisions to optimize yields.
Blue River Technology’s See & Spray
Use: Uses computer vision to identify and spray weeds.
Benefit: Reduces herbicide usage by up to 90%.
Microsoft FarmBeats
Use: Collects data from sensors and drones to provide insights into on-farm conditions.
Benefit: Affordable solution for small-scale farmers.
Climate FieldView (by Bayer)
Use: Monitors field conditions and provides recommendations for planting and harvesting.
Benefit: Increases productivity and reduces costs.
Agrible
Use: Predicts weather and provides crop management advice.
Benefit: Helps farmers plan better and reduce risks.
Granular
Use: Tracks farm operations and financial performance.
Benefit: Improves farm management and profitability.
FarmLogs
Use: Monitors crop health and provides alerts for potential issues.
Benefit: Early detection of problems saves crops.
Prospera
Use: Uses AI to analyze visual data from fields.
Benefit: Provides actionable insights for better crop management.
Taranis
Use: High-resolution imaging for pest and disease detection.
Benefit: Prevents crop losses and improves yields.
CropX
Use: Soil sensors and analytics for irrigation management.
Benefit: Optimizes water usage and improves crop health.
People also ask
Yes, Machine Learning (ML) is transforming agriculture by enabling data-driven decision-making, increasing efficiency, and improving yields. ML can be applied in various agricultural domains, including:
2️⃣ Crop Yield Prediction
3️⃣ Pest & Disease Detection
4️⃣ Automated Irrigation Systems
5️⃣ Supply Chain Optimization
6️⃣ Livestock Monitoring
Yes, machine learning can significantly improve farm management by enabling data-driven decision-making. It helps in precision farming, automated irrigation, pest detection, and yield prediction.
by precision farming, data-driven, pest and disease detection, irrigation automation, automation-based tractors etc.
Machine learning improves crop health by enabling early disease detection, optimized resource use, and real-time monitoring. Here’s how:
1️⃣ Disease & Pest Detection – AI-powered image recognition detects infections early, allowing timely intervention.
2️⃣ Precision Irrigation & Fertilization – ML analyzes soil moisture and nutrient levels to optimize water and fertilizer use.
3️⃣ Weather Prediction – AI forecasts climate changes, helping farmers protect crops from extreme conditions.
Conclusion-
The future scope of machine learning in agriculture is immense. From precision farming to climate resilience, ML is set to revolutionize the way we grow food. Farmers can increase productivity, reduce costs, and contribute to a sustainable future by adopting these technologies. However, it is crucial to address challenges like data accessibility and affordability to ensure that the benefits of ML reach every farmer.
Author Bio-
Khumesh is an agriculture technology expert with 5 years of experience in the agriculture field. He has worked on AI projects and is passionate about using technology to solve real-world problems. Connect with khumesh on LinkedIn or visit agrigramodaya.com for more insights.
References-
Read latest post-
📚 Cotton Corporation of India syllabus 2025 Notification out for 147 post
“Are you preparing for the Cotton Corporation of India (CCI)...
Read More📋 MPPSC Food Safety Officer Syllabus 2025 Latest Exam Pattern, Salary & Preparation Guide!
Suppose you are preparing for the MPPSC Food Safety Officer...
Read MoreZero Budget Natural Farming 2025: Real Story of a BSc Agri Student Who Said No to Job
1. Introduction: Zero Budget Natural Farming in 2025—A true success...
Read MoreWhat is bsc agriculture
BSc Agriculture means bachelor of science and birthplace of India...
Read More
A Udyam registration certificate is necessary to obtain loans from banks and benefits provided under various schemes to MSMEs. – The Udyam registration certifies that an enterprise is covered under the MSME category